IHMC researcher using cognitive science, novel language understanding methods to harness AI’s power
Dr. Ian Perera started his research career by exploring the question of whether computers could become more intelligent and helpful assistants by learning the way children do.
If the IHMC Research Scientist can make it work, the implications could be wide-ranging and substantial.
Since joining IHMC in 2013, Perera has worked on numerous military and government projects using novel language understanding methods and cognitive science on problems from de-escalating heated social media conversations to improving trust between human and AI team members and more. His work is featured in the latest edition of the IHMC newsletter, available now.
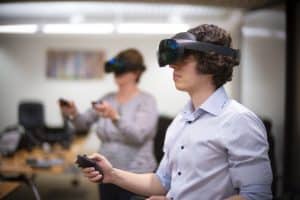
Research Scientist Dr. Ian Perera’s work at IHMC’s Ocala campus includes artificial intelligence, cognitive science and more.
The popular culture’s focus on headlines claiming AI can perform complicated tasks and will soon replace our workforce misses something critical, Perera says.
“This technology is uncritically learning associations between words and concepts, and mimicking behaviors of people online that isn’t always grounded in reality,” Perera says.
While there is some similarity to the powerful associative learning connections that children use when they encounter something new, imbuing AI with a more critical and exploratory approach could lead to a powerful capability with a transformative influence on human decision-making.
It could benefit warfighters in the heat of battle, cool the overheated world of social media commentary, and even improve the long-term health and well-being of military personnel.
While artificial intelligence has a fever-grip on the public imagination, Perera has delved into the true possibilities and limitations of this discipline. And here’s a hint: It’s not about supplanting human intellect.
“The goal is to take our knowledge about how we learn things and use it to inform our models so that they get a better understanding more quickly,” Perera said. “We are looking at strategies to augment associative learning that could be translated to artificial intelligence.”
Typical AI training involves a massive data dump into the system to “teach it,” but that creates an AI that is only as trustworthy as the data it has been fed. Perera has worked to find ways to use language to teach machine learning systems and minimize flaws such as implicit bias.
Having trust in an AI-teammate’s decision is critical to the successful integration of the technology into human decision-making, especially for military operators and others who could rely upon such data to make life-or-death decisions.
“What we were looking at is, can we make sure that an explanation is encoded into the system so that when it makes a decision, you can see if it seems like a logical consequence of what the system is deciding,” Perera says.
Perera’s current work includes a project for the U.S. Navy where AI is responsible not only for finding irregularities or unexpected events, but also for providing the user with multiple possible explanations given the context. For example, multiple ships may be stationary nearby because they are waiting out a storm, or aspects of their behavior may point to illicit activity.
The applications are wider ranging than warfighting, however.
One such project, Civil Sanctuary, had the goal of engaging in social media communities with automated dialogue agents to help with content moderation.
And if there is any place where there is room for improvement, it is the world of online discourse.
Civil Sanctuary aimed to spot language that would indicate when people in an online forum are crossing the line from disagreeing to becoming toxic.
“We wanted to see if we could say something about the emotions being conveyed or the moral foundation” of the comments, Perera said.
Keeping a human in the loop of content moderation is ideal, but the volume of content to moderate makes it nearly impossible for humans to keep up. AI could be helpful in this, especially if it can sense the tone and emotional meaning beneath the words.
Perera’s modeling took several things into account to try to gauge when a moderator gets involved in the interplay among commentors. He also worked on modeling how the community responds to certain emotions.
“We can pick up on it before the human would and before it can do more damage, and say to the human in the loop, ‘Hey, this may be something you want to look at,’” he said.
Countering implicit bias
In AI research, human judgement is seen as the “ground truth”or the “correct” answer. However, we know that everyone has implicit biases that affect how they take in and respond to information. Even if a belief is grounded in fact or good intentions, the nature of the expression of that belief can shut down constructive discourse.
Yet there may be a way forward, aided by AI.
“Sometimes, if you’re aware of the bias, then you can start to see what you might want to change about that,” Perera said.
Towards this end, Perera and his team developed an “echo-chamber burster” — a method to analyze language and suggest refinements that would make a user’s comment on social media more constructive, even de-escalating potentially toxic or insulting discourse.
“We wanted to see if we could change how a comment is phrased to come to some common ground and reduce the toxicity,” Perera said. “Can we make the sentiment less angry and generate language that people can just engage with that’s not highly emotionally charged.”
The work coming out of this project presents a new vision of the potential for generative AI – one that creates new opportunities for bridging ideological differences by reframing communication in terms of the beliefs and ideals of the person or group across that divide.
Studying trust calibration
Another of Perera’s efforts looked at a co-training methodology to calibrate trust building in human-machine teams. At its core, this method sees the human and the AI agent train together, each learning as they go along.
That has included building a user interface that allows the human and machine partners in a team to navigate tasks and avoid obstacles together.
This co-training model gives the human and the AI team members each more feedback about their performance than a traditional model.
“We see (it) as being open with your strengths and weaknesses,” Perera says.
The findings so far suggest that team performance improves with such an approach.
“It makes the AI aware of its limitations and then encourages the human user to consider where AI can be applied most effectively,” he said. “In fact, we found that in this task, having an AI that was open about its capabilities and suggested delegations created a more effectively performing team than simply improving the AI’s accuracy by 20 percent.
“When we talk about improving systems that are used by people in decision making, this result shows us we should be focusing more on the human element, rather than chasing percentage points of accuracy.”
Ongoing work that Perera is part of includes looking at virtual reality tools that may help identify the impacts of mild and sub concussive traumatic brain injury before the condition might be clinically diagnosed. These are instances in which symptoms are difficult for even humans to identify but can have long-term consequences.
“When I think about the potential of AI, I’m not as focused on how we can do tasks as well as humans. I instead look at opportunities for AI to tell us something about ourselves or the world that we might miss as humans,” Perera says. “To do that, we need to turn a critical eye to ourselves and teach AI to do the same for its judgement.”
IHMC is a not-for-profit research institute of the Florida University System where researchers pioneer science and technology aimed at leveraging and extending human capabilities. IHMC researchers and staff collaborate extensively with the government, industry and academia to help develop breakthrough technologies. IHMC research partners have included: DARPA, the National Science Foundation, NASA, Army, Navy, Air Force, National Institutes of Health, IBM, Microsoft, Honda, Boeing, Lockheed, and many others.
Latest News
- Air Force Academy’s Cadet Summer Research Program interns find home at IHMC
- STEM-Talk: Charles Serhan, expert on specialized pro-resolving mediators, talks inflammation
- IHMC partners with California-based research institute to take aim at the psychology of cyber attackers
- STEM-Talk: Dave Feldman on the Citizen Science Foundation, ketogenic diet research
- New biomedical research complex opens IHMC’s next chapter
- Triumph Gulf Coast funding will help bolster research capabilities at Pensacola campus
- IHMC Robert Griffin represents the America on IEEE Technical Committee
- STEM-Talk: Alessio Fasano on the roots of, treatments for celiac disease
- IHMC hosts the IGNITE event for the NATO Innovation Continuum